Today, business choices cannot be based on intuition and personal opinions. Businesses that use data as a strategic asset are more competitive, run more efficiently, and predict the future more accurately. Earlier, executives’ experience and the paucity of information formed the basis for decisions. Today, massive amounts of information – from users’ behavior to trends in the market – allow organizations to make right, accurate, and most importantly, predictable decisions.
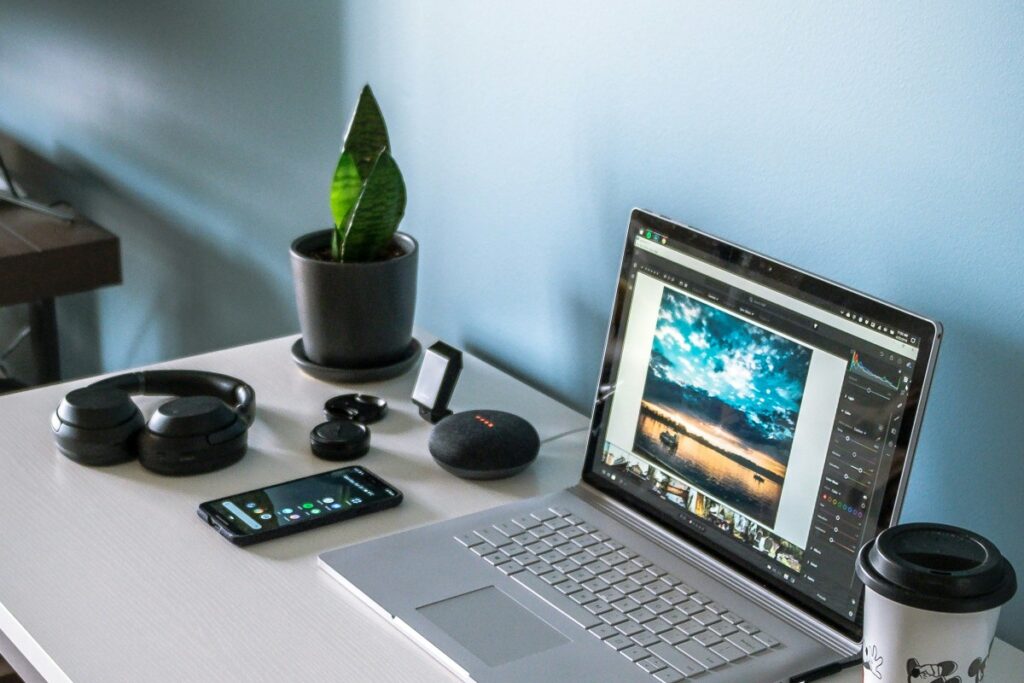
Big Data Strategies have been the pillar of digital transformation. It is used in marketing, supply chain, financial analysis, healthcare, and nearly every area of enterprise. But data is just the first step. Success comes from processing it well, analyzing it, and employing it to formulate powerful strategies.
In this article from Celadonsoft, we will observe how organizations use data to make decisions, what technologies and tools assist them in doing so, and what are the problems that may arise while adopting data-driven strategies.
Understanding the Data-Driven Decision-Making Principle
Experience and intuition are no longer the only sources of decision-making for companies nowadays. Data-driven companies use analytics, scalability of forecasting, and artificial intelligence to minimize uncertainty and maximize the efficacy of strategies. This practice, as well as Cybersecurity Best Practices for 2025, is known as Data-Driven Decision-Making (DDDM) – data-driven decision-making.
What Is Data-Driven Decision-Making (DDDM)?
DDDM is a methodology where all important business decisions are taken based on data analysis and not guesswork, speculation, or opinion. DDDM allows businesses to:
- Make unbiased decisions. Data provides you with a real picture of what is happening, not an interpretation.
- Reduce risks. Analytics allows you to foresee possible issues and respond before they occur.
- Enhance efficiency. Computer-aided data processing helps you identify the best solutions sooner.
- Optimize resources. Businesses are able to allocate budget, individuals and technical resources more efficiently.
Why Is Transitioning to Data-Driven So Important?
Markets are changing quickly, and business needs to make decisions based on data that makes a difference. For example, retailers use insights into sales and customer behavior to personalize promotions and improve conversion. Banking institutions scan transactions to predict risk. Production companies optimize supply chains based on data on demand and availability of resources.
Using Big Data Strategies decision-making is not a trend, but it is necessary for companies to achieve sustainable development and competitiveness. All people who keep using data-driven methods reach closer to reality with greater insights, react in a timely manner to market variation, and achieve their objectives successfully.
Critical Steps in Implementing Data-Driven Approaches
To effectively utilize big data for decision-making, it is significant to formulate a clear-cut strategy that includes several critical steps. It is not just an exercise of grinding information – it is a simple method of business management that requires proper goal setting, collecting quality information, analyzing it, and intelligently using the intelligence derived.
1. Defining Goals and Setting Business Objectives
All the strategies start by identifying what we want to do. Big Data Strategies provide valuable data, but unless we have clear business objectives defined, it’s worthless.
Good examples of setting goals:
- E-commerce: Get maximum conversions on the site through personalized discounts.
- Finance: Get maximum risk assessment processes done with predictive analytics.
- Logistics: Get minimum transportation costs by predicting demand.
It must be of paramount importance that objectives are clear, quantifiable and attainable (SMART rule). And data analysis will derive actual business worth only then.
2. Gathering and Uniting Pertinent Information
Following the definition of the objectives, one must choose the sources of information. They may either be company internal systems (CRM, ERP, logs of user behavior) or external (social media, open databases, IoT sensors).
The essential questions to be answered during this phase are:
- What is the information required in order to realize your objectives?
- What are the sources from which it may be obtained?
- How can one verify the consistency and validity of the information gathered?
Real case study: in retail, when forecasting demand, it is necessary to consider not only sales data, but also outside influences such as seasonality, economic trends, and even the weather.
It is necessary to organize one data warehouse (Data Warehouse, Data Lake) and ensure integration with analytical tools.
3. Data Analysis and Interpretation
Raw data is meaningless. Statistical methods, machine learning, and data visualization are used here.
General analysis tools:
- BI tools (Power BI, Tableau) – simple to use for reporting and visualization.
- Programming languages (R, Python) – allow for comprehensive analytics and machine learning model building.
- Big Data platforms (Apache Spark, Hadoop) – optimal for processing lots of unstructured data.
4. Decision-Making and Strategy Implementation
Once the data has been analyzed, the most important thing is to act. It is paramount that data-driven decisions are embedded in real business processes.
How to optimally implement insights?
- Automate decision-making (for example, dynamic pricing for e-commerce).
- Create an A/B testing workflow and test hypotheses before implementing global changes.
- Train the team – analytics should not only be accessible to technical experts, but also to decision makers.
Big Data Working Technologies and Tools
Contemporary technologies and tools are necessary for data-driven decision-making. There are many solutions available today that allow businesses to store, process and collect large amounts of data efficiently, let’s look at the principal tool categories.
Data Processing and Storage Platforms
Hadoop is one of the most widespread distributed big data processing systems. It guarantees data storage and processing in server clusters.
- Apache Spark – a powerful high-speed data processing system supporting both machine learning and real-time analytics.
- Google BigQuery – cloud storage with the ability to execute SQL queries at high speed on terabytes of data.
- Amazon Redshift – a database for analytics of high-performance structured data.
Analytical Tools and Visualization
- Tableau is a simple-to-use data visualization platform that allows business users to analyze with analytics without the need to code.
- Power BI – a Microsoft offering that interoperates with other products in the platform, enabling simple data analysis.
- Google Data Studio – a free tool for creating interactive dashboards and reports from various sources of data.
- Python (pandas, matplotlib, seaborn) – high-level data processing, analysis and visualization libraries, widely used by data analysts.
Using these tools, companies are not just capable of effectively managing large sets of data, but also of transforming them into useful business solutions.
Real-Life Examples of Good Implementation of Data-Driven Strategies
Let’s analyze how leading corporations are using big data to maximize business performance and make better-informed decisions.
Marketing and Personalization
Internet marketing and online shopping companies are using data to provide personalized user experiences. Netflix, for example, uses a user’s viewing behavior to suggest what they’ll most likely be watching. Amazon uses machine-learning algorithms for pricing dynamically and recommendation.
Supply Chain Management
Logistics companies and retailers use big data to forecast demand and enhance supply chains. Walmart, for example, examines weather patterns, consumers’ behavior, and other factors in a bid to predict ahead of time what would be trendy in various locations.
Financial Sector
Banks and investment firms use data analysis to quantify risk and detect fraud. Mastercard, for example, uses machine-learning algorithms in a bid to detect suspicious purchases in real-time.
These all serve to demonstrate how information is a powerful tool that, if applied correctly, can greatly improve business performance.
Challenges and Risks in Using Data-Driven Methods
Although the advantages of working with data are clear, companies have a set of challenges to consider when using data-driven methods.
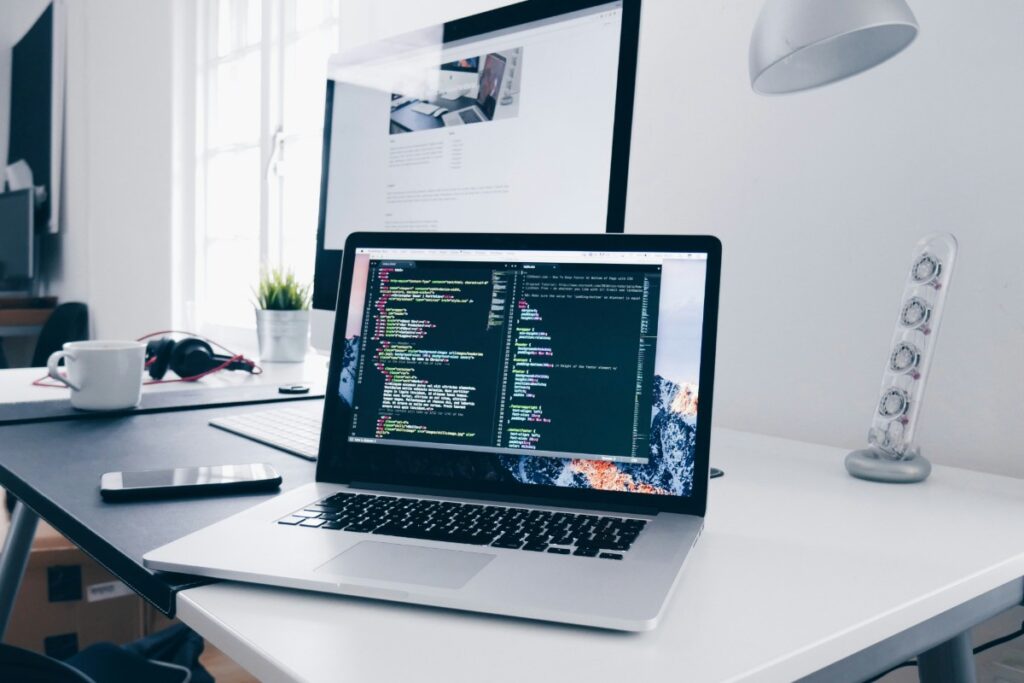
Data Quality and Reliability
Handling missing or low quality data is one of the key problems. Even the most sophisticated algorithms will not be able to make accurate predictions if data is incorrect, contains duplicates or is old. To address this issue, companies like Celadonsoft are implementing data cleansing, standardization and data quality management procedures.
Ethical and Legal Considerations
During an era when there is increased surveillance of personal data, organizations must remember legislation such as GDPR (European Data Protection Regulation) and CCPA (California Data Protection Act). A violation of such legislation can not only result in fines, but also undermine customer trust.
Resistance to Change in the Organization
Not everybody is prepared to fully depend on data in decision-making. There are times when people prefer to believe in experience and intuition rather than analytical data. To counter this problem, companies like Celadonsoft are investing in employee training and establishing a data culture.